SLIDE – Satellite Images Prediction with Deep Learning
Winning project from the 1st Open Call funded by the AI4Copernicus Project
Companies: Solaïs / Transvalor
Countries: France | Domain: Energy
An interview with the winners – Thomas Carriere, representing the SLIDE project
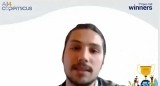
Q: What was your motivation for creating this proposal?
A: The two partners of this project, Solaïs and Transvalor, were both interested in integrating Artificial Intelligence in their algorithms for a good time. Although satellite data was already available through Transvalor, both weather data, computing resources and Cloud infrastructure to disseminate the work were lacking. The AI4Copernicus call aligned perfectly with these needs and we hope to produce a nice product to promote Copernicus data.
Q: Which is the most critical impact (societal or other) that your project could make (if you could name one) ?
A: The most critical impact is the increase in performance for solar power forecasting in a few hours. Forecasting solar power based on satellite imagery typically has trouble extrapolating cloud motions for this forecast horizon. Increasing this forecasting performance is particularly important for the management of energy systems and the safe operation of the power grid.
Q: Considering the recent funding received through the AI4Copernicus Open Calls, do you have any plans for further development of your idea?
A: One of the main ideas made possible thanks to the AI4Copernicus Open Call funding is the inclusion of weather forecasts data coming from Copernicus in addition to satellite images, in order to infer physical drivers of cloud motions. Without this call, both the data and the computing infrastructure would have been very difficult to obtain.
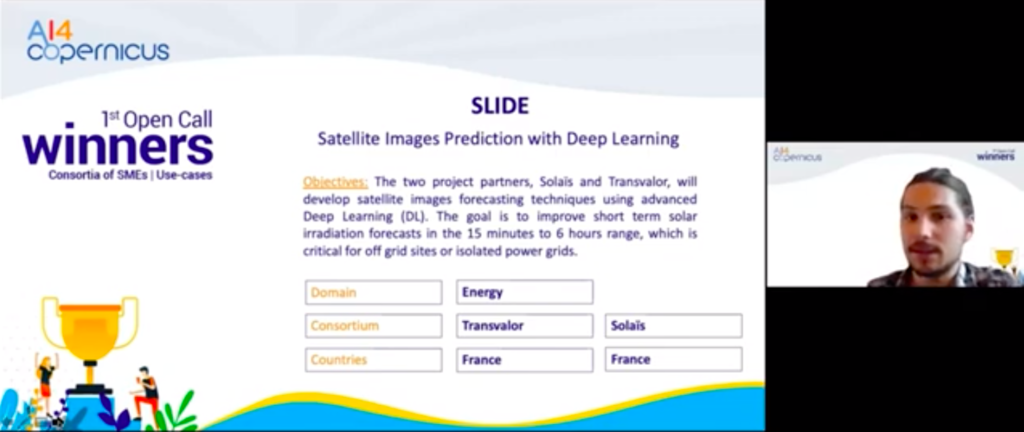
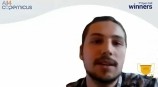
Abstract of the project: The two project partners, Solaïs and Transvalor, will develop satellite images forecasting techniques using advanced Deep Learning (DL). The goal is to improve short term solar irradiation forecasts in the 15 minutes to 6 hours range, which is critical for off grid sites or isolated power grids. Innovative Deep Learning methods will be tested and validated against standard (persistence) and Machine Learning methods on past data. A special care will be taken to represent rapid solar irradiation changes called ramps. Criterion for images forecast evaluation will be studied to validate the forecast service over a large area. The result will be downstream services accessible through the AI4EU marketplace and running on a DIAS. Users will be able to provide geographical coordinates for which they need a forecast, and the request will be executed on DIAS hardware then output to the user.
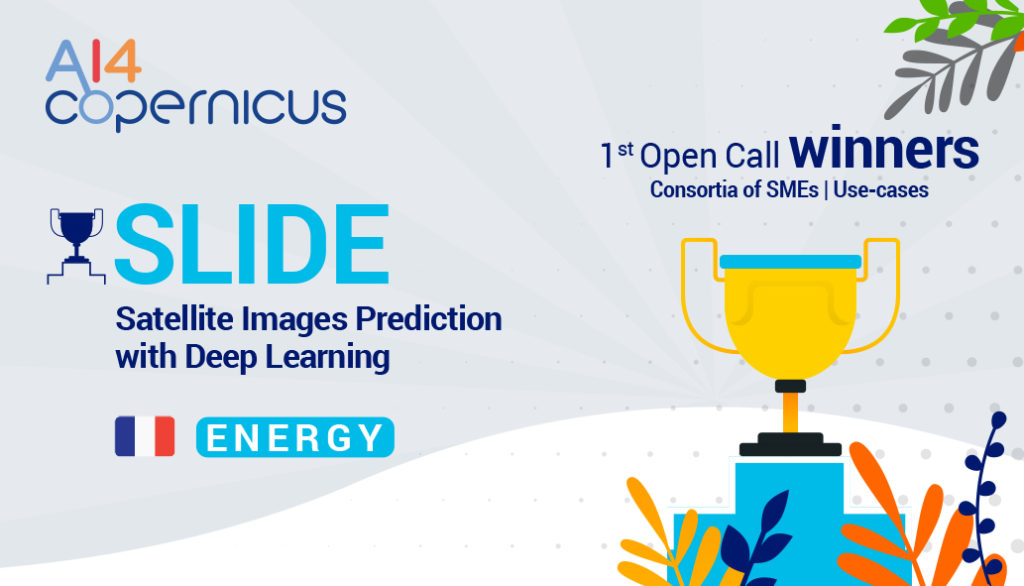
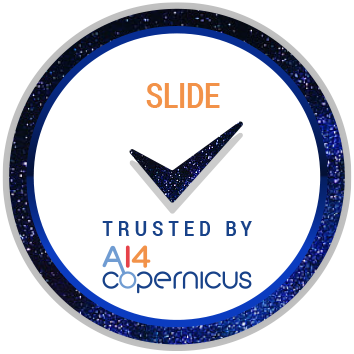